Table of Contents
ToggleMachine learning stands at the forefront of this paradigm, offering a robust framework for dissecting complex search engine data patterns. This technology enables granular analysis of SEO metrics, enhancing the strategic decision-making process with its capacity for predictive analytics and adaptive learning.
For professionals seeking mastery over SEO dynamics, machine learning provides an indispensable toolset, facilitating the automation of data collection, the sophistication of content optimization, and the strategic refinement of backlink profiles.
By employing machine learning algorithms, marketers can gain a deeper understanding of competitor benchmarks and ROI metrics, ensuring a data-driven approach that aligns with the fluidity of search algorithms and user behaviors. Thus, machine learning transforms SEO performance tracking into a more precise, intelligent, and results-oriented endeavor.
Key Takeaways
- Machine learning enables a granular understanding of SEO dynamics by parsing vast datasets.
- Understanding SEO metrics such as search volume, CTR, and bounce rate is crucial for enhanced visibility and engagement.
- Machine learning facilitates the identification of emerging trends and market shifts in SEO.
- Data collection automation through machine learning algorithms evolves SEO performance tracking beyond traditional practices.
Understanding Machine Learning
Machine learning, a branch of artificial intelligence, leverages algorithms to analyze and interpret complex data, enhancing SEO performance tracking processes. This computational paradigm employs morphological elements to structure data points into meaningful patterns, essential for deciphering search engine behaviors. By applying a linguistic approach, machine learning can dissect the morphology of search queries, aligning them with relevant industry measurements such as click-through rates and keyword rankings. These insights allow for the optimization of web content, ensuring adherence to unique industry standards.
Through the use of predictive analytics, machine learning provides a technical scaffold for SEO strategies, forecasting potential shifts in user engagement and search relevancy. By parsing vast datasets, machine learning algorithms enable a granular understanding of SEO dynamics, empowering practitioners to fine-tune their approaches with precision.
SEO Metrics Fundamentals
In the context of semantic linguistics, morphology imparts structure to keywords, while industry measurements provide quantifiable data about their performance. Product brands invoke assessment through search volume and brand mentions, illustrating market presence and consumer awareness.
Metric | Description |
---|---|
Search Volume | Measures the number of searches for a keyword. |
Click-Through Rate (CTR) | Indicates the percentage of users who click on a listing after viewing it. |
Bounce Rate | Reflects the percentage of visitors who leave the site after viewing only one page. |
Mastery in SEO necessitates an analytical approach to these metrics, informing strategies for enhanced visibility and engagement.
Machine Learning in SEO
Machine learning incorporation into SEO strategies allows for precise analysis of metrics like search volume, click-through rate, and bounce rate, thereby improving the prediction and comprehension of user behavior patterns. Through the application of advanced algorithms, SEO professionals can dissect vast arrays of data, revealing insights that drive strategic decision-making. The integration of machine learning facilitates:
- Identification of emerging trends and market shifts.
- Optimization of content for relevance and engagement.
- Refinement of keyword strategies based on predictive search behaviors.
- Customization of user experiences through personalization tactics.
- Evaluation of link quality and the impact on domain authority.
This analytical approach empowers SEO experts to craft data-driven strategies with an elevated level of accuracy, ensuring that their efforts align with the nuanced demands of search engine algorithms and user expectations.
Data Collection Automation
Harnessing machine learning algorithms, SEO performance tracking evolves through the automation of data collection, enabling real-time analysis and agile responses to search engine dynamics. This systematic approach to data aggregation transcends traditional practices and is underpinned by the precision of morphological analysis and the robustness of local points of interest within industry measurements. The integration of machine learning into SEO data collection facilitates an advanced understanding of product brands, trends, and performance metrics.
Morphology | Local Points of Interest | Industry Measurements |
---|---|---|
Keyword Stemming | Search Queries | Click-Through Rates |
Lexical Tagging | Competitor Analysis | Bounce Rates |
Syntax Parsing | User Engagement | Conversion Rates |
Predictive Analytics Application
Predictive analytics, empowered by machine learning, significantly enhances SEO strategies by forecasting future trends based on current data patterns.
- Morphology informs the structuring of keywords for optimal search engine recognition.
- Product Brands leverage predictions to align marketing strategies with anticipated search queries.
- Industry measurements track the performance impact of SEO activities pre-emptively.
- Local points of interest get highlighted in predictive local SEO, enhancing visibility for regional searches.
- Morphology aids in understanding user intent, and improving content relevance and engagement.
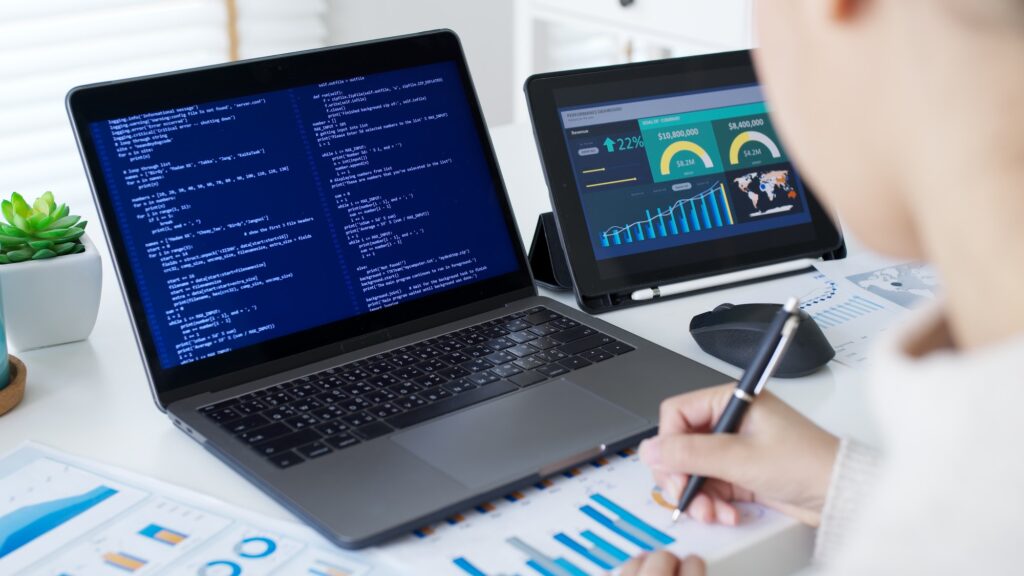
Applying these insights drives a technical and analytical approach to SEO, focusing on the linguistic and pragmatic aspects that shape search engine algorithms. This methodology not only anticipates the direction of user interest but also adapts to the evolving language of search queries, providing a competitive edge in the digital marketplace.
Algorithmic Updates Adaptation
Machine learning equips SEO strategists with the agility to swiftly respond to search engine algorithmic changes, maintaining website rankings and ensuring content relevance. This technology parses vast sets of search data, identifying patterns that signal alterations in algorithms. It models potential impacts on search result pages, enabling preemptive optimization strategies.
By leveraging historical data, machine learning algorithms predict which SEO tactics will align with the search engine’s revised criteria, morphologically adapting to the linguistic environment of queries and content. This continuous adaptation is critical, as search engines like Google can update their algorithms several times a year, each with the potential to pivotally shift industry measurements and website traffic flows. Thus, machine learning provides a robust framework for SEO resilience in a landscape of perpetual evolution.
User Experience Enhancement
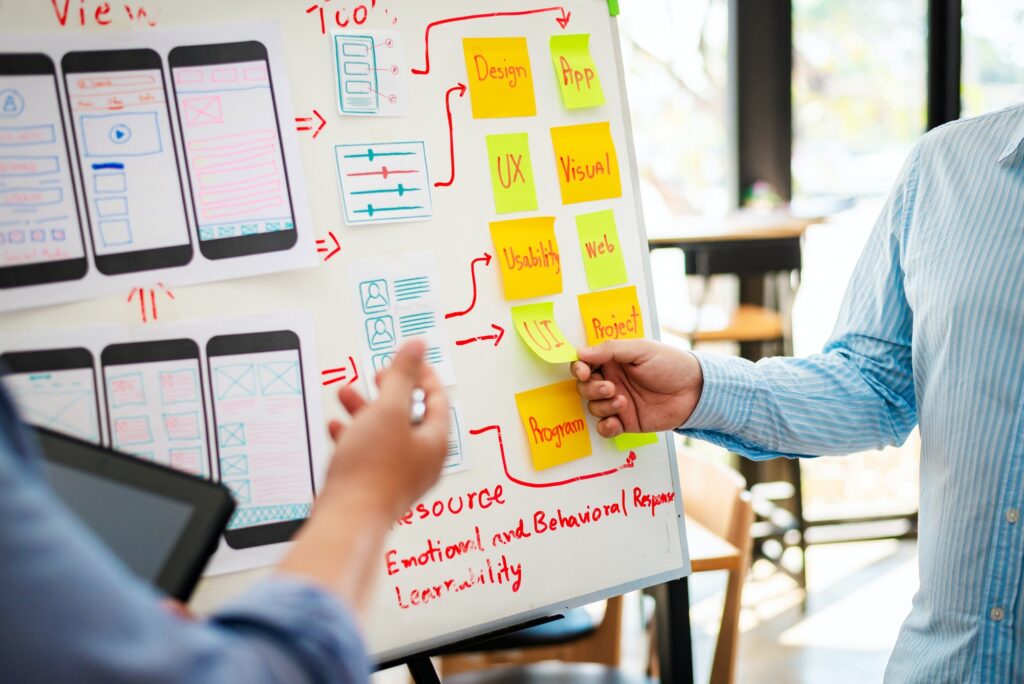
Through the application of machine learning algorithms, one can discern patterns that indicate optimal user engagement and satisfaction. This intelligence drives SEO experts to fine-tune their websites, ensuring that they not only align with search engine algorithms but also provide an exemplary user experience. Key elements include:
- Page Load Speed: A swift response to user queries.
- Navigation Ease: Intuitive and straightforward site architecture.
- Content Relevance: Precise and valuable information delivery.
- Mobile Optimization: Seamless cross-device compatibility.
- Interactive Elements: Engaging features that encourage user participation.
Real-Time Decision-Making
The integration of machine learning algorithms with SEO tools allows for the continuous monitoring of morphological changes in search patterns, enabling the dynamic adjustment of content to maintain relevance. Machine learning’s capability to process vast sets of industry measurements and user interactions translates into granular insights.
These insights inform SEO specialists on local points of interest, product brand performance, and linguistic pragmatics affecting search engine visibility. The result is an SEO strategy that responds in real-time to market and language shifts, keeping websites consistently at the forefront of search engine result pages, thereby ensuring sustained organic traffic and engagement.
Content Optimization Strategies
While machine learning enhances real-time decision-making in SEO, it also significantly refines content optimization strategies by analyzing user engagement and search queries to tailor content that aligns with evolving linguistic trends and search behaviors.
- Predictive analysis of search patterns identifies morphological shifts, allowing for preemptive content adjustments.
- User engagement metrics inform syntactic and pragmatic alterations to enhance clarity and intention.
- Linguistic profiling maps local points of interest to regional linguistic variations, optimizing local SEO.
- Entity recognition algorithms extract industry-specific terminology, ensuring technical accuracy.
- Machine learning models assess product brand resonance within market segments, guiding focused content creation.
These strategies underscore machine learning’s pivotal role in sculpting bespoke content ecosystems, where linguistic precision meets user intent, fostering an authoritative digital presence.
Backlink Profile Analysis
Given that a robust backlink profile is a cornerstone of search engine optimization, machine learning plays a crucial role in analyzing the quality and relevance of inbound links to enhance a website’s authority and rankings. Through meticulous scrutiny, machine learning algorithms can discern patterns and assess the impact of various backlink attributes on SEO outcomes. By processing large datasets, machine learning offers a nuanced understanding of backlink profiles, enabling SEO professionals to make informed decisions.
Metric | Relevance to SEO |
---|---|
Link Quality | Determines authority |
Anchor Text | Affects keyword relevance |
Source Domain Authority | Influences link equity |
Link Type (Dofollow/Nofollow) | Impacts link value |
This approach ensures a data-driven strategy in cultivating a backlink ecosystem that is both diverse and aligned with industry measurements, thereby optimizing search visibility and performance.
Competitor Benchmarking Insights
Machine learning elevates competitor benchmarking by precisely analyzing peers’ SEO strategies to identify areas for improvement and opportunities for ranking advancements. By leveraging complex algorithms, businesses can gain actionable insights into:
- Morphology and industry measurements of competitors’ keyword optimization.
- Local points of interest influencing search engine results page (SERP) positioning.
- Product brands and their search visibility against market benchmarks.
- Backlink profiles and their contribution to domain authority.
- Content strategy effectiveness through engagement metrics comparison.
This analytical approach provides a technical roadmap to calibrate SEO efforts more efficiently. Machine learning does not merely track competitors’ performance but uncovers the technical intricacies of their success, enabling a strategic response that is informed, precise, and potent.
ROI Measurement and Growth
We now turn our attention to the critical aspect of ROI Measurement and Growth in the realm of SEO Performance Tracking, where machine learning quantifies the financial impact of SEO strategies. Tracking SEO performance involves analyzing a vast array of data points. Machine learning algorithms excel in dissecting these data sets, identifying patterns, and providing actionable insights. By attributing conversions and revenue to specific SEO initiatives, businesses can calculate a clear return on investment. Growth, in this context, is evidenced by an upward trend in these financial metrics over time. A precise measure of ROI enables companies to refine their SEO strategies, allocate budgets more efficiently, and ultimately, enhance their market position by driving more value from their SEO efforts.
Frequently Asked Questions
How Does Machine Learning Assist in Identifying and Prioritizing Technical SEO Issues That May Not Be Apparent Through Traditional Analytics?
Machine learning algorithms excel at detecting patterns and anomalies, enabling the identification of technical SEO issues beyond the scope of traditional analytics, thus prioritizing fixes for optimal website performance and search engine visibility.
In What Ways Can Machine Learning Contribute to Understanding and Predicting Consumer Search Behavior Changes Over Time?
Machine learning can analyze vast datasets to detect patterns, predict search behavior trends, and enable proactive adaptation to consumer needs, thus enhancing the relevance and effectiveness of content presented to users.
What Is the Role of Machine Learning in Assessing the Impact of Voice Search and Smart Devices on SEO Strategies?
Machine learning evaluates voice search and smart device data, providing insights into SEO strategy optimization by analyzing user interaction patterns and linguistic variations to enhance search engine visibility and user experience.
How Does Machine Learning Influence the Creation and Adjustment of SEO Strategies for Local Businesses Versus Global Enterprises?
Machine learning enables nuanced strategy customization for local businesses and global enterprises by analyzing large datasets to identify trends and inform adjustments specific to regional or international market dynamics.
Can Machine Learning Help in Detecting Fraudulent or Spammy Backlink Activities, and How Does It Ensure the Protection of a Website’s SEO Integrity?
Machine learning algorithms can effectively identify fraudulent backlinks, enhancing a website’s SEO integrity by recognizing patterns indicative of spammy activities, thus safeguarding its search engine ranking position and digital reputation.
Conclusion
The integration of machine learning into SEO performance tracking stands as a significant advancement in digital marketing analytics. By harnessing the capabilities of sophisticated algorithms, businesses can automate data collection, employ predictive analytics, refine content strategies, analyze backlink profiles, and gain competitive insights with unprecedented precision. This fusion of technology and strategy not only enhances the measurement of ROI but also propels the continuous growth and optimization of online visibility in an ever-changing digital landscape.